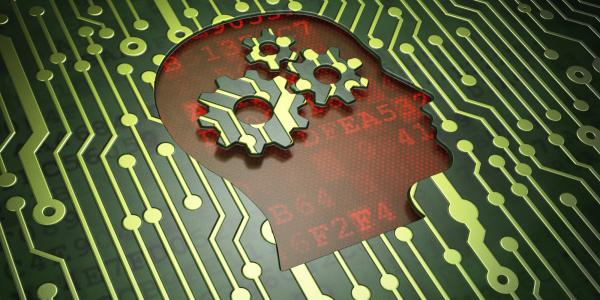
NEW ONLINE DATABINGE, FRIDAY’S STARTING 12:30PM
We have re-booted Databinge as an online meeting to tackle coding and new approaches to data analysis. The team will be online via slack and will breakout in to Zoom meetings to discuss points of interest in more detail. Contact a tutor via email (see bios below) for slack invites and zoom addresses. We recommend labs prep OSF projects in order to share data and code. Info on using OSF can be found here.
TUTOR BIOS
ABHIJIT CHINCHANI (Djavad Mowafaghian Centre for Brain Health)
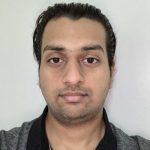
Abhijit is an electrical engineer by training, with a strong drive to understand the brain. He uses his quantitative skills in signal processing and machine learning to solve convoluted problems in cognitive computational neuroscience. He is currently a graduate student in the Department of Bioinformatics at UBC. Under the guidance of Dr. Todd Woodward, he is investigating the physiological and behavioral effects of transcranial alternating current stimulation (tACS). His work involves using brain imaging techniques like EEG, MEG, and fMRI and developing analysis workflows to process the data. These include preprocessing, dimensionality reduction of the imaging data, and prediction. He is also involved in designing psychophysical experiments and analyzing behavioral data. Although most of his coding experience is in MATLAB, he also has some experience with Python, C, and Java. Abhijit’s email
Analysis techniques/tools used:
- Statistical control of artifacts in dense array EEG/MEG studies (SCADS) – MATLAB
- Unsupervised dimensionality reduction techniques like PCA and ICA – MATLAB
- Supervised dimensionality reduction
- Partial least squares-discriminant analysis (PLS-DA) – MATLAB
- Denoising Source Separation (DSS) using joint decorrelation – Noise toolbox, MATLAB
- Gaussian Process Factor Analysis (GPFA) – MATLAB
- Constrained PCA – MATLAB
- Multitaper spectral analysis – Chronux Toolbox, MATLAB
- Multidimensional signal detection model – MATLAB
- EEGLAB, Fieldtrip – MATLAB
- Psychtoolbox – MATLAB
- Machine learning models like – SVM, KNN, decision trees, neural nets – MATLAB, Python
PANKAJ GUPTA (Djavad Mowafaghian Centre for Brain Health)
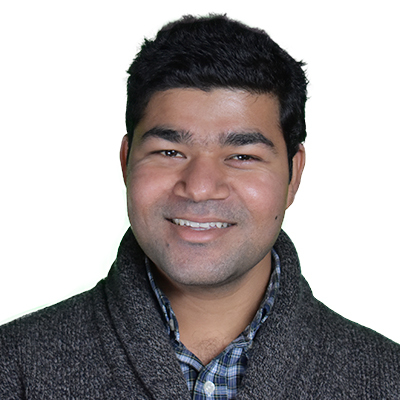
Pankaj is a graduate student in Neuroscience at UBC. He is interested in building computational and quantitative tools to understand Brain functions and novel interventions in neurological disorders. He did Bachelor’s in Computer Science and MSc. in Interactive Technologies and had worked in industry as a software developer as well as a research engineer in academia, prior to joining UBC. He has experience working with open-source tools (OpenCV, EEGLab, OpenGL) and has contributed to some, such as HOMER. He has experience working with programming languages (Python, Matlab, C#, C++, shell scripts) on Windows and Linux platforms. At UBC, he works with Dr. Timothy Murphy on a project aimed to study how to induce neuroplasticity in neo-cortex in mice. Calcium imaging is his primary tool to record neural activity but he has some experience with EEG and NIRS data as well. Apart from these, he is interested in working with and learning about other imaging techniques such as TMS, fMRI and ECoG. Pankaj’s email
Analysis techniques/tools used:
- Programming Languages: Python, Matlab, C#, C++, VC++ (Win32API), C (on POSIX compliant OS), Python, SQL, HTML, CSS
- Raspberry-Pi programming with hardware devices and GPIO (Python)
- DeepLabCut for tracking (Python)
- Audio feedback generation and mapping to a range of inputs ( Python)
- Image processing using OpenCV (C++, Python)
- Augmented Reality and visualizations using OpenGL (C++)
- Electroencephalogram data processing using EEGLab (Matlab)
- Near-Infrared Spectroscopy data analysis using Homer toolkit (Matlab)
- Microsoft Kinect (Depth Sensor) programming (C++, C#)
- Nintendo Wiimote Programming (C#)
- Microsoft .NET (C++, C#)
- Virtualization (C#, C++)
- Computer Networking (C++, C#, Python, Matlab)
ADRIAN LINDSAY (Djavad Mowafaghian Centre for Brain Health)
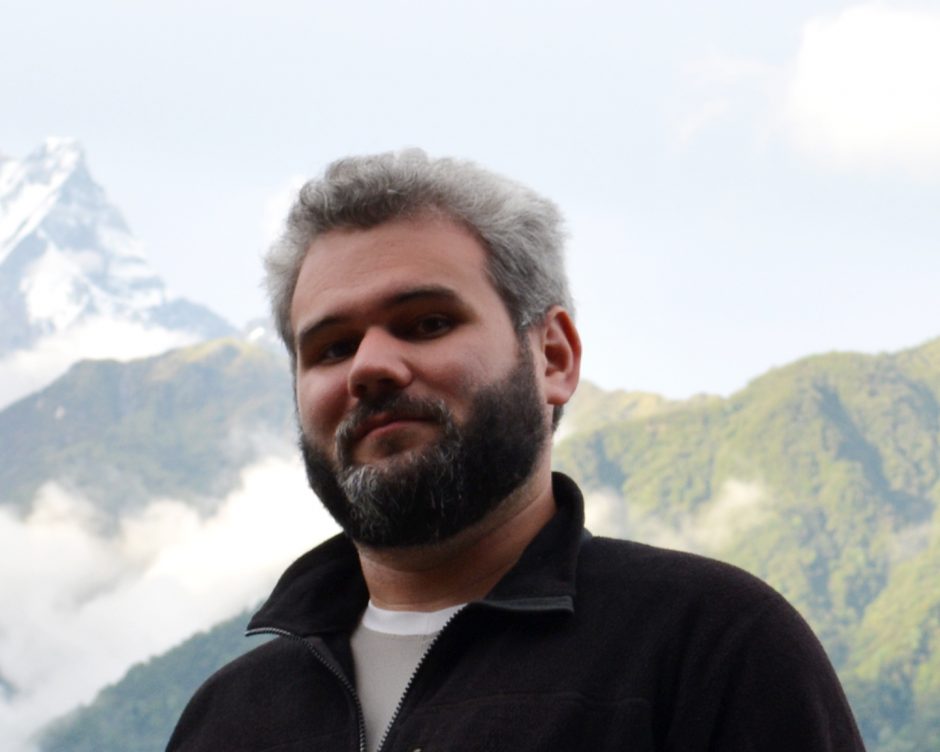
Adrian has a passion for the future of technology, in particular the future of our efforts to understand human intelligence, artificial intelligence and the interface between humans and machines. He has combined his mixed background in computer and animal science, and is currently pursuing a PhD in computational neuroscience under the guidance of Dr. Jeremy Seamans at the UBC DMCBH. His work involves developing computational models of the function of neural ensembles, their relation to behaviour, and applications of machine learning algorithms in neural encoding, computer vision, and other multi-data-stream analysis paradigms. In addition, he regularly works as a TA for the department of Computer Science, and has experience working in and teaching a variety of coding languages, including Python, MATLAB, Java, Javascript, C and C++. Adrian’s email
Analysis techniques/tools used:
- Artificial Neural networks for unsupervised, supervised, and semi-supervised learning (regression and classification)
- Convolution NN models for computer vision and neural encoding
- Recurrent NN for neural encoding and behavioural prediction
- Phase space analysis for signal processing and behavioural classification
- Global (PCA, ICA, etc.) and local (T-SNE) dimensionality reduction and data preprocessing methods
- Unsupervised clustering (mixture models, watershed transforms, etc) for data exploration and discovery
- Ensemble and bagging machine learning models for classification and regression (SVM, Random Forests, etc)
- DeepLabCut – automated tracking of features from video: used to track features on lab animals during experiments
- Motion Mapper – automated behaviour classification from video for fruit flies
- B-SOID – automated behaviour classification in open field mouse experiments
KAITLIN SULLIVAN (Life Sciences Institute)
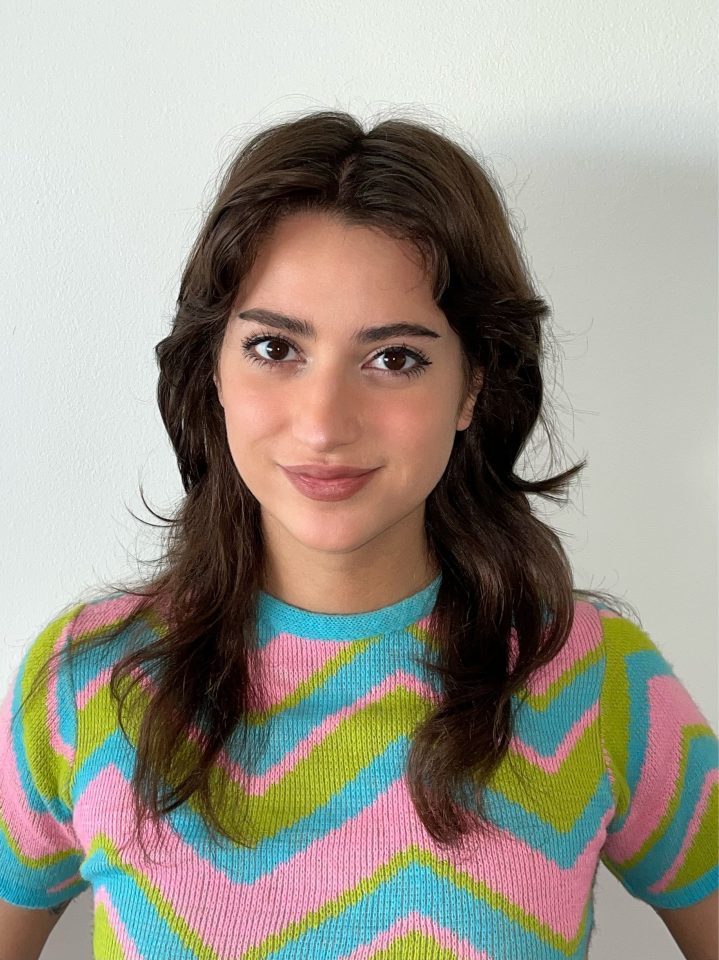
Kaitlin is a Neuroscience PhD candidate with a background in cognitive science. Her research is focussed on identifying precise molecules and cell types participating in fear memory encoding and retrieval. In particular, her work involves the use of single cell transcriptomic techniques such as single cell RNA sequencing (scRNA-seq) and multiplexed fluorescent in situ hybridization (mFISH). As such, she is experienced in using R for the integration, dimensionality reduction, clustering, and identification of differential gene expression in scRNA-seq datasets. She furthermore is well-versed in the open-sourced image analysis software FIJI’s macro language (a Java-like language) and has developed user-friendly computational tools for mFISH analysis on this platform. Kaitlin furthermore has experience developing R Shiny Apps for sharing of simple, code-free analysis. In addition to her work, Kaitlin assists in the teaching of an R workshop for CAPS students at UBC alongside the DBC Tutorial. While her main language is R, she also has familiarity with Python and Java.
Analysis techniques/tools used:
- Basic image processing – FIJI (IJM macro language)
- Registration and Segmentation – FIJI (IJM macro language)
- ImageJ macro creation – FIJI (IJM macro language)
- Dimensionality Reduction (PCA, tSNE, UMAP) – R
- Clustering (kmeans, hierarchical, Louvain) – R
- scRNA-seq Analysis (with Seurat) – R
- Visium Analysis (with Seurat) – R
- R Package creation – R
- Shiny App development – R